Can Pictionary and Minecraft test AI models’ ingenuity?
- November 5, 2024
- Posted by: chuckb
- Category: TC Artificial Intelligence
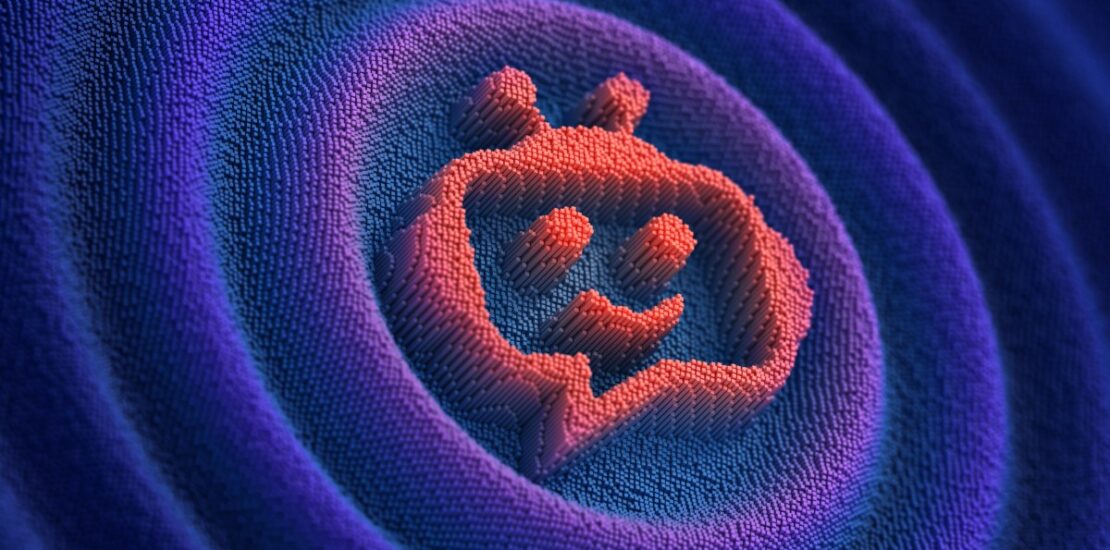
The exploration of AI benchmarks has been a hot topic, with many existing assessments failing to provide meaningful insights into the true capabilities of artificial intelligence systems. Traditional benchmarks often revolve around rote memorization or trivial topics unrelated to practical applications for the majority of users. In response to these limitations, some AI enthusiasts are turning to games as a more engaging and revealing way to evaluate AI problem-solving skills.
One prominent figure in this movement is Paul Calcraft, a freelance AI developer who created an innovative app that enables two AI models to engage in a Pictionary-like game. In this setup, one model doodles while the other attempts to guess what the doodle represents. Calcraft envisioned this project as not only fun but also as an intriguing challenge that encourages the models to think beyond the confines of their training data. He drew inspiration from fellow programmer Simon Willison, who had previously tasked AI models with rendering complex visuals, like a pelican on a bicycle. Calcraft aimed to develop a benchmark that defies traditional methodologies, explicitly creating a situation where the models cannot simply memorize specific answers or patterns encountered during their training.
Adding to the gaming-based benchmarking dialogue, 16-year-old Adonis Singh has developed a tool named Mcbench, which utilizes the popular game Minecraft to evaluate an AI’s design capabilities. Singh believes that Minecraft promotes resourcefulness and autonomy among the AI models, making it significantly less constrained than traditional benchmarks. He asserts that the format of Minecraft is less saturated and allows for more creative exploration of AI skills.
While the concept of using games to benchmark AI is not new—dating back to Claude Shannon’s musings in 1949 about chess as a benchmark for intelligent software—recent advancements have shifted focus onto large language models (LLMs). These models, which can analyze various data formats, are now being subjected to games to scrutinize their logical reasoning abilities. The current landscape is rich with a variety of LLMs, such as Gemini, Claude, and GPT-4o, each presenting unique behaviors in interaction, adding a layer of complexity to evaluating their performance.
Calcraft points out that LLMs are notoriously sensitive to how questions are phrased, making their performance unpredictable. In contrast, games introduce a visually engaging and intuitive framework for evaluating decision-making capabilities. Matthew Guzdial, an AI researcher, emphasizes that games provide a different avenue for understanding AI behavior compared to text-based benchmarks, offering varied simplifications of reality that focus on decision-making.
The application of Pictionary as a measure of AI reasoning aligns closely with the principles of Generative Adversarial Networks (GANs), where a model creates images that another model judges. Calcraft observes that Pictionary can gauge an LLM’s comprehension of elements like shapes, colors, and spatial relationships. He acknowledges, however, that while Pictionary serves as an interesting exploration of spatial understanding, it is ultimately a simplistic scenario that may not adequately reflect real-world reasoning.
Similarly, Singh holds that Minecraft allows for a comprehensive evaluation of LLM reasoning capabilities, claiming that his assessments correlate well with his overall trust in the models’ reasoning abilities. However, not all experts share this enthusiasm. Mike Cook, a research fellow at Queen Mary University specializing in AI, argues that Minecraft does not possess unique qualities that distinguish it as a specialized AI testbed compared to other games. He observes that the game’s superficial resemblances to the real world might contribute to its appeal among non-gaming people, but insists that it does not significantly differ from other gaming environments in evaluating problem-solving capabilities.
Cook further notes that even the most advanced AI models often struggle to adapt to new contexts or tasks they haven’t encountered before. He acknowledges that while Minecraft has noteworthy features, such as weak reward signals and a procedurally generated environment that presents unpredictable challenges, it is no more representative of real-world tasks than other games like Fortnite or World of Warcraft.
Despite differing perspectives regarding the effectiveness of Minecraft and Pictionary as benchmarks, the fascination with these gaming contexts persists. Observing LLMs attempting to construct complex structures in Minecraft or creatively doodling in Pictionary provides an engaging glimpse into how AI might approach tasks that require conceptual understanding and critical thinking.
Ultimately, using games as a benchmark for AI evaluation might not yield a definitive methodology; however, it sparks interest in diverse approaches to understanding the capabilities of these advanced models. The playful engagement of games can bridge gaps between complex machine learning concepts and intuitive understanding, offering a platform where creativity and reasoning can be intuitively observed and assessed. As the exploration of AI continues to evolve, the gaming landscape will likely play an increasingly significant role in shaping how we comprehend and evaluate artificial intelligence’s capabilities.