How phishing pages admit to being LLM-made
- November 4, 2024
- Posted by: chuckb
- Category: Securelist
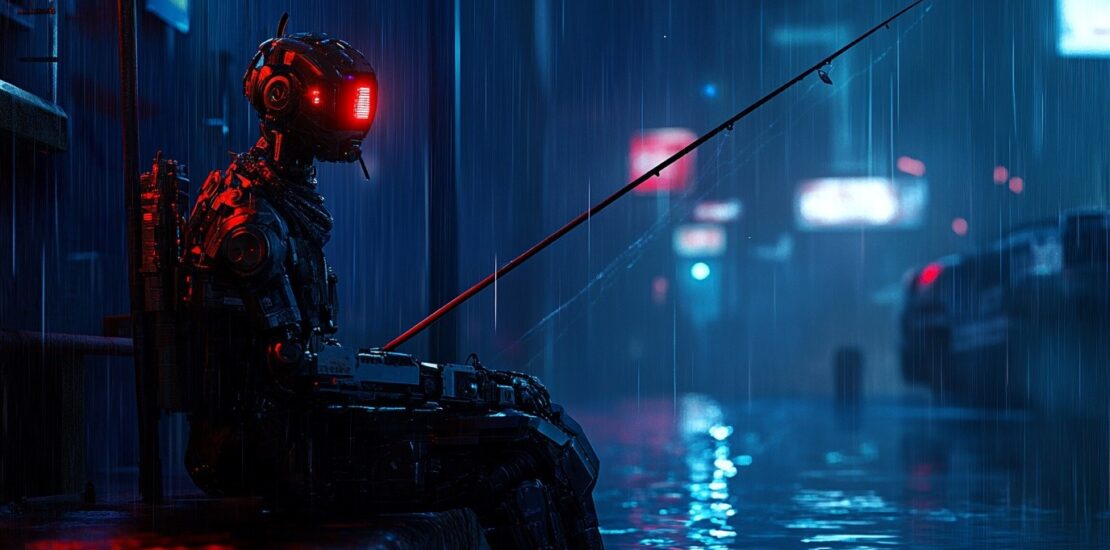
The rise of large language models (LLMs) has notably contributed to a phenomenon known as ‘capability uplift’, wherein employees with minimal experience can significantly enhance their performance, particularly in fields like information security. This is crucial given the escalating risks posed by cyberattacks, which are increasingly sophisticated and cost-efficient. Attackers are now leveraging LLMs to create fraudulent content that simulates legitimate websites, enabling them to conduct classic phishing schemes and impersonate reputable brands offering false discounts.
LLMs facilitate the automated generation of multiple web pages with unique, high-quality content—far surpassing the capabilities of traditional synonymizers—allowing scammers to evade detection by relying on rules grounded in specific phrases. This kind of detection now necessitates advanced systems capable of analyzing metadata, page structures, or employing machine learning techniques to identify anomalies more effectively. However, LLMs are not infallible; inconsistencies in their responses can create identifiable artifacts indicating the use of these models in malicious activities.
Certain distinctive markers, such as the frequent apology phrases used by LLMs when refusing to create certain types of content, serve as clear indicators of their involvement in generating fraudulent materials. For example, in a campaign targeting cryptocurrency users, webpages often feature LLMs apologizing before providing incomplete or unsatisfactory responses. This pattern of expressing apologies or declining instructions reveals limitations in automation, suggesting either a low level of control in the content generation process or specific parameters set within the automated pipeline.
Moreover, artifacts appear throughout not only the textual content but also the metadata associated with these fraudulent pages. Instances where LLMs state their inability to generate useful content without specific keywords serve as a telltale sign of LLM involvement. These irregularities extend to various web formats, from messages indicating limits on response length to incomplete outputs because the model exceeded its character constraints, further exposing the artificial nature of the generated texts.
Common phrases associated with LLMs, such as expressions of knowledge cutoffs, provide insight into the timeframe of the scam websites’ content. For instance, many scam pages acknowledge their lack of updated information after a specified date, giving clues about when the content was created and the duration of the associated campaigns. Identifying these phrases not only aids in recognizing LLM usage but also assists in estimating the recency of the scam’s operational timeline.
Additionally, certain vocabulary choices, like the word “delve” and expressions about the “ever-evolving” landscape of cryptocurrency, frequently emerge in LLM-generated content and can be seen as indicative of such automation. Scammers often adopt these phrases, along with stylistic peculiarities like Unicode obfuscation, to circumvent detection measures designed to identify generative patterns.
As automation technologies evolve, LLMs’ strengths and weaknesses are becoming more discernible, and while their use in fraud is increasing, the blur between human-written and machine-generated content is likely to deepen. Currently, the identification of LLM-generated fraud relies heavily on detecting specific patterns or phrases, which could easily shift as scammers adapt their methods. Hence, the pursuit of reliable methodologies for automatic detection of LLM-text will continue to be a complex challenge.
To effectively combat phishing scams—whether crafted by human perpetration or machine generation—modern security solutions are deemed necessary. These solutions should integrate text analysis, metadata evaluation, and other attributes to provide comprehensive protection against evolving fraudulent tactics, ensuring that cybersecurity at large can keep pace with the sophisticated tools made available by LLM advancements.
In conclusion, the interaction between LLMs and cybercriminal activities highlights an urgent need for enhanced security measures as LLM technology becomes more prevalent within the context of online fraud. Continued research and development in tracking and mitigating these threats will be vital as the landscape of cybersecurity and digital fraud continues to evolve.