MIT debuts a large language model-inspired method for teaching robots new skills
- November 4, 2024
- Posted by: chuckb
- Category: TC Artificial Intelligence
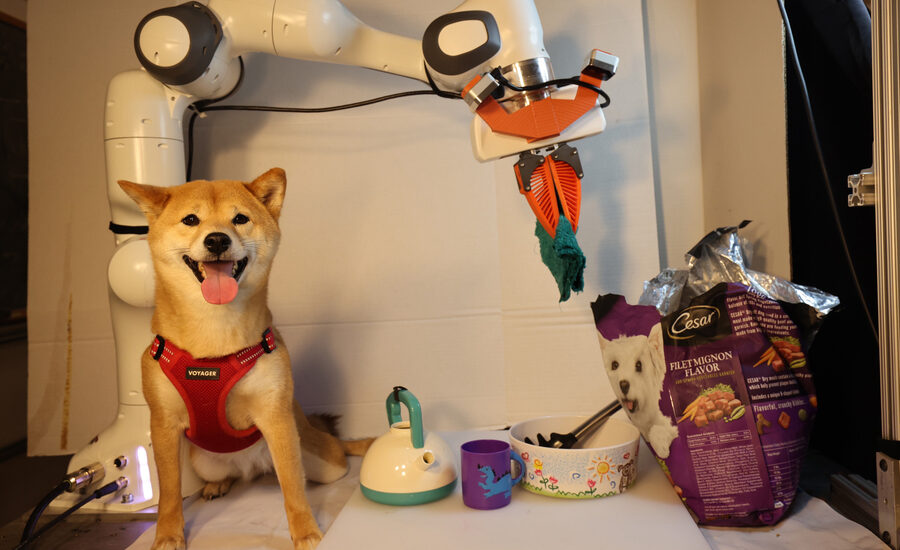
This week, researchers at MIT introduced a groundbreaking approach to training robotic systems that contrasts sharply with traditional methods reliant on specialized datasets. Instead of utilizing narrow data for teaching robots specific tasks, the new methodology draws inspiration from large language models (LLMs), epitomized by systems like GPT-4, by leveraging vast amounts of diverse information.
One major limitation of conventional robotic training methods, particularly imitation learning—which involves a robot learning tasks by copying a demonstrator—is their failure to adapt to varying conditions. When small challenges arise, such as changes in lighting, the environment, or unexpected obstacles, robots often struggle to respond effectively due to the lack of sufficient data to tackle these new situations.
To address this challenge, the MIT team envisioned an approach analogous to the data-rich environments in which LLMs thrive. “In the language domain, the data are all just sentences,” notes Lirui Wang, the lead author of the research. “In robotics, given all the heterogeneity in the data, if you want to pretrain in a similar manner, we need a different architecture.”
In response, the researchers developed a novel architecture known as Heterogeneous Pretrained Transformers (HPT). This new model is designed to integrate insights from various sensors along with data collected from different environments, effectively broadening the range of information available during training. By employing transformers, a robust machine learning technique, they can unify this heterogeneous data into coherent training models. The effectiveness of these models appears to correlate with the scale of the transformer used; larger transformers yield improved outputs.
As part of this innovative framework, users can provide specific details about their robot’s design, configuration, and the tasks they need assistance with. This interactive element emphasizes flexibility and user-driven customization, a significant advantage for robotics applications.
David Held, an associate professor at Carnegie Mellon University and a collaborator in the research, expressed ambitious aspirations regarding this work. He envisions a future where a “universal robot brain” could be downloaded and implemented in robots with no need for further training, effectively democratizing advanced robotic capabilities. He acknowledges that while this research is currently in its nascent stages, sustained efforts may lead to significant advancements in robotic policies, mirroring the progression observed in the realm of large language models.
This innovative research endeavor has been partially funded by the Toyota Research Institute (TRI), which has demonstrated a keen interest in accelerating robot training methods. At last year’s TechCrunch Disrupt event, TRI introduced a method allowing robots to be trained overnight. Recently, TRI has formed a notable partnership aimed at integrating its robot learning research with the advanced hardware offered by Boston Dynamics, further hinting at the practical applications of this research in real-world robotics.
Overall, this new methodology represents a significant paradigm shift in the training of autonomous systems, aiming to equip robots with the adaptability and versatility required to navigate complex and dynamic environments. Plans for future development indicate a concerted push toward a more accessible and intelligent robotics solution, akin to the transformative effects seen with large language models in artificial intelligence. As research advances, the team hopes to refine these methods and achieve a breakthrough that will enhance robotic capabilities across various applications.